top of page

Between January 1990 and December 1999, the Search and Rescue (SAR) team in Yosemite Valley performed 1912 SAR missions, assisting 2327 individuals and recording 2077 injuries and illnesses. The average mission required 12 SAR personnel and cost $4400. Despite their heroic efforts, 112 fatalities occurred throughout the 10-year study period, yielding a SAR case fatality rate of 4.8% [1]. Over 100 missions took place in hard-to-reach areas such as El Capitan and Half Dome, where helicopter rescue may be impossible due to the steepness of the terrain. In some cases, the rescue time greatly exceeded the average of 5 hours due to the challenge of locating victims with limited knowledge of their geographic position, surrounding hazards or state of health.
To aid in these efforts, advances in sensing, high-performance computing and low SWaP (size, weight and power) unmanned aerial systems (UAS) are enabling higher squad-level intelligence and situational awareness. Still, the majority of UAS require user-designated waypoints to plan their autonomous flight, leaving the operator to make critical decisions with limited knowledge. Improvements in online decision-making and situational awareness would greatly reduce the odds of fatality in these stressful situations by providing a basis for more informative reasoning and planning in the air. This level of analysis would support robust planning for such missions without placing a cognitive burden on the SAR operationalists. As an aerospace engineer who has witnessed these dangerous circumstances first-hand through rock climbing, I feel morally responsible for helping to improve these technologies. The following timeline provides an overview of my progress - even in this early stage of development.
Stay tuned for updates, open-source flight code and an occasional blurb on my thoughts from the field!
​
[1] E. Hung, D. Townes, "Search and Rescue in Yosemite National Park: A 10-Year Review," Wilderness & Environmental Medicine, 18, pp. 111-117, 2007.
- Nate Osikowicz
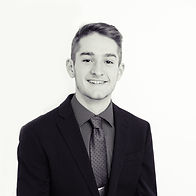.jpg)
System Overview
Mission Timeline
​Feb. 2020
​Apr. 2020
​Mar. 2020
​Jul. 2020
First HITL Test on the 1DOF Test Stand
​Notable Progress: ​
-
Accel. angles from the ADXL345 close the loop on PWM motor commands via simple PID control
-
To improve performance, the ADXL will be replaced with an MPU6050 using a Complementary Filter
Jul.
2020

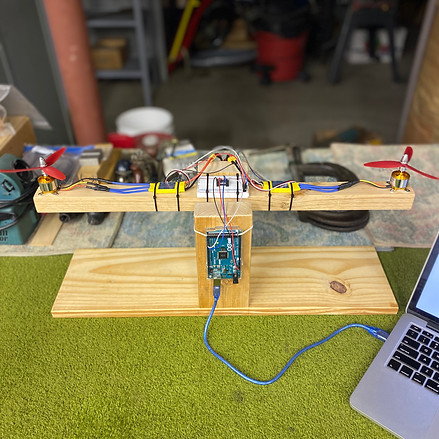
HITL
Apr.
2020
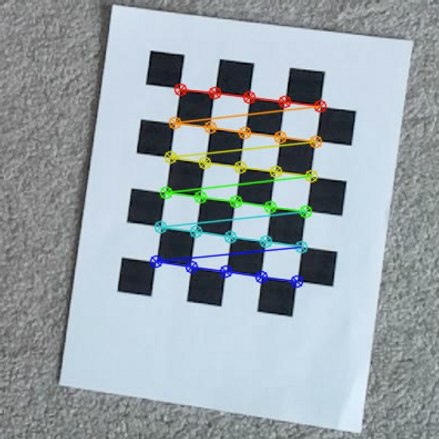
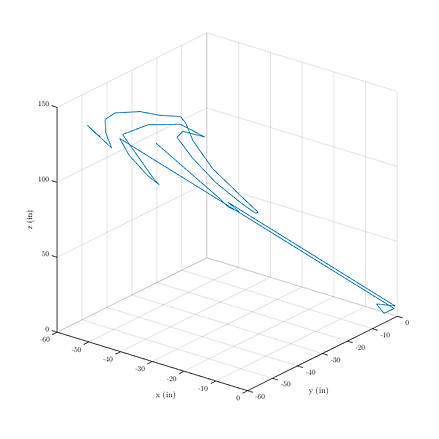

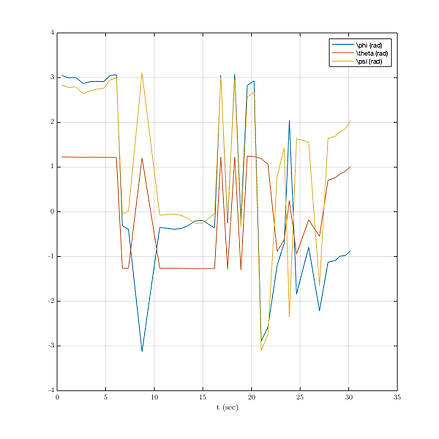
Computer Vision Localization over the Landing Pad
​Notable Progress: ​
-
The vehicle successfully determined its pose using camera observations during a hand-carry
-
Our flight controller uses a Raspberry Pi Cam and the openCV library to estimate attitude and position
-
Future tests will integrate camera observations into the EKF for a precision landing
CV
Gρ spread its wings! See the vehicle's first flight.
​Notable Progress: ​
-
The Gρ quadrotor maintained a stable hover for close to 1 minute in the PSU Hammond Building
-
Our flight controller is running the latest version of ArduCopter in Stabilize mode
-
Flight data is telemetered down to a Windows machine running the Mission Planner software
Mar.
2020
firstflight
Feb.
2020
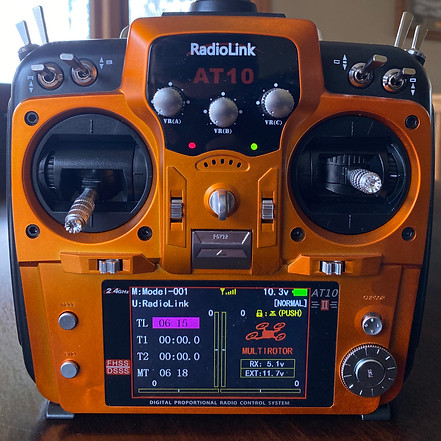
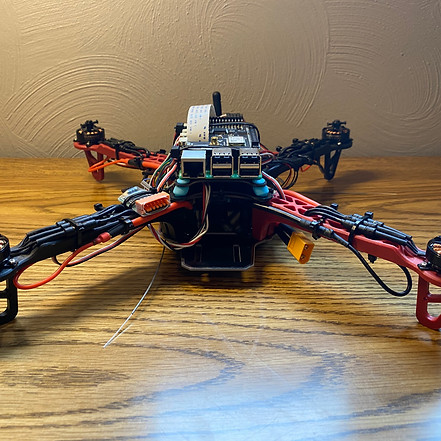
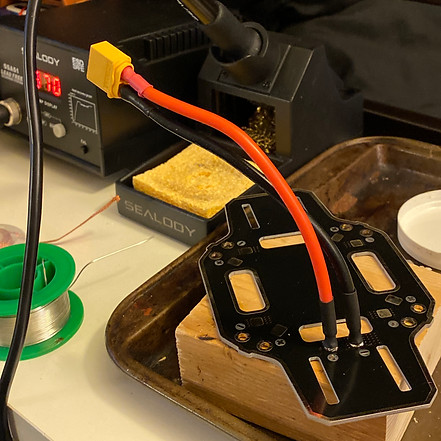

Meet Gρ! A lightweight UAS for GPS-denied Autonomy
​Notable Progress: ​
-
Weighing in at close to 600 grams, Gρ is a lightweight UAS that was named after our capstone team
-
The quadrotor is equipped with a Raspberry Pi Cam, lidar and dual IMUs on a RPi 3b with Navio 2 hat
-
For now, Gρ will fly using off-the-shelf flight software until we get our algorithms together
grho
bottom of page